enterprise risk management for resilient supply chain
Mitigating risks via Enterprise Risk Management tools
Risk management in the supply chain is undoubtfully a very complex topic, requiring a structured approach to effectively navigate the myriad uncertainties and potential disruptions.
Without a systematic framework in place, identifying, assessing, and mitigating risks can be daunting tasks fraught with ambiguity. However, leveraging and embracing advanced methodologies and tools based on probabilistic modeling offers a structured means of quantifying and monitoring risks.
By integrating probabilistic techniques with data-driven insights, organizations can gain a deeper understanding of the probabilistic dependencies and interrelationships within the supply chain ecosystem. This enables them to proactively identify vulnerabilities, assess the likelihood and impact of various risk events, and implement targeted mitigation strategies to enhance resilience and mitigate the potential impact of disruptions on supply chain operations.
Bayesian Networks for effective risk monitoring
In the labyrinth of decision-making, where uncertainties lurk at every corner, Enterprise Risk Management tools, mainly based on Bayesian Networks, emerge as guiding beacons, illuminating pathways through the darkness of ambiguity. At their core lies a mathematical schema that intertwines probability theory with graphical representations, enabling the modeling, inference, and understanding of complex systems.
Conditional Probability:
Central to Bayesian networks is conditional probability, a cornerstone of probability theory. It quantifies the likelihood of an event occurring given the occurrence of another event. Mathematically, it’s expressed as:
P(A∣B)= P(A∩B)/P(B)
Here, P(A∣B) denotes the probability of event A occurring given that event B has occurred, P(A∩B) represents the joint probability of both events A and B occurring, and P(B) is the probability of event B occurring.
Graphical Representation:
Bayesian networks are epitomized by directed acyclic graphs (DAGs), where nodes represent variables or events. Directed edges between nodes denote probabilistic dependencies or causal relationships. Each node is associated with a conditional probability distribution, quantifying the likelihood of the node’s value given the values of its parent nodes.
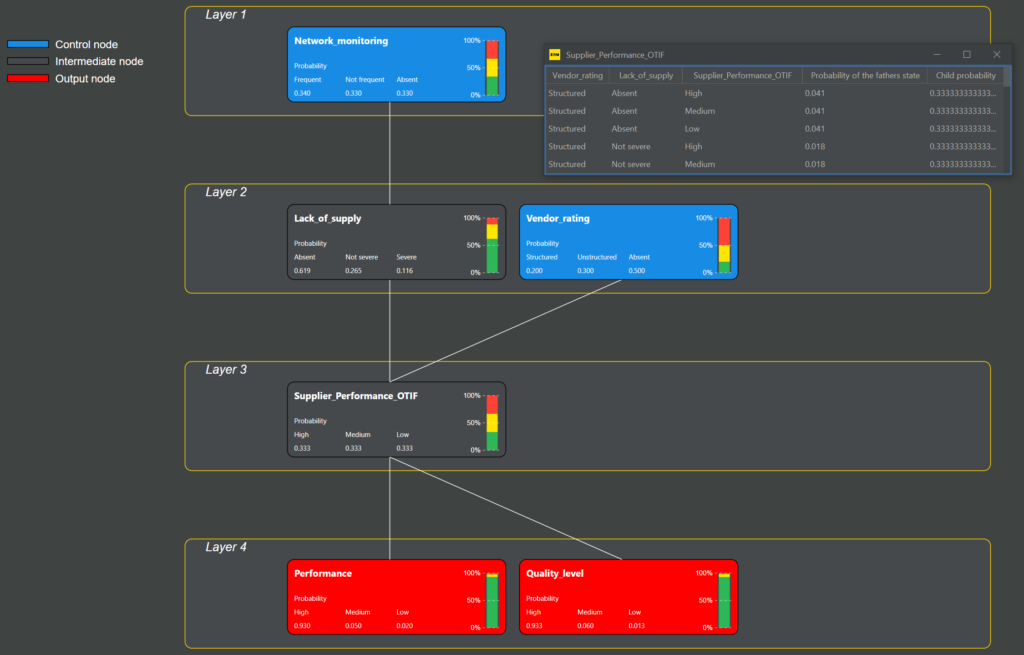
Conditional Probability Tables (CPTs):
Conditional probability tables are the scaffolding upon which Bayesian networks are built. They capture the conditional probabilities of each node given its parent nodes’ values. For nodes with parents, the CPT specifies the probability distribution of the node’s possible states conditioned on the parent nodes’ states.
Bayes’ Theorem:
Bayes’ theorem, a fundamental principle underpinning Bayesian networks, facilitates the updating of probabilities based on new evidence. Mathematically, it’s expressed as:
P(A∣B)=P(B∣A)×P(A)/P(B)
Here, P(A∣B) is the posterior probability of A given B, P(B∣A) is the likelihood of B given A, and P(A) and P(B) are the prior probabilities of A and B, respectively.
The mathematical schema characterizing Bayesian networks embodies the fusion of probability theory, graphical representations, and inferential methodologies. As organizations traverse the landscapes of uncertainty, Bayesian networks stand as formidable allies, guiding them through the complexities of decision-making and illuminating pathways to resilience and innovation. In an era defined by uncertainty, Bayesian networks serve as lighthouses, casting rays of clarity amidst the fog of ambiguity.
Main applications of Bayesian Networks
Bayesian networks empower diverse applications, from machine learning and artificial intelligence to risk management and diagnostic systems. In this article, we will explore two main usage of such methodology: Supply chain risk management and Predictive maintenance.
In the first case the DAG is used to explore the impact of risks on the whole supply chain and simulate plausible combination of events (i.e. a port closure and lack of containers).
In the second, DAGs support diagnostic maintenance by leveraging probabilistic inference to identify the root causes of failures and prognostic maintenance by predicting future machinery health.
Both the two cases deal with risk management, but at a different level of abstraction.
1. ERM for the supply chain
In supply chain risk management, Bayesian networks offer probabilistic modeling, scenario analysis, and decision support, enabling organizations to navigate uncertainties with confidence. They aid in building anti-fragile supply chains by identifying vulnerabilities, dynamically managing risks, and optimizing resilience strategies.
Risks, in the supply chain, can derive from many different sources, here shortly summarized.
Supplier Reliability: In a manufacturing supply chain, a company relies on multiple suppliers to provide raw materials. However, the reliability of each supplier varies, and disruptions in the supply chain can occur if a critical supplier fails to deliver on time. A Bayesian network can be constructed to assess the reliability of each supplier based on historical performance data, lead times, geographical location, and other relevant factors. By incorporating conditional probabilities, the network can calculate the likelihood of a supplier meeting delivery deadlines or encountering disruptions, enabling the company to prioritize suppliers and implement contingency plans accordingly.
Demand Forecasting and Inventory Management: Accurate demand forecasting is essential for optimizing inventory levels and ensuring customer satisfaction. A Bayesian network can be used to model the probabilistic relationships between various factors influencing demand, such as seasonality, marketing campaigns, economic indicators, and competitor activities. By incorporating historical sales data and external variables, the network can generate probabilistic forecasts of future demand. These forecasts can then be used to optimize inventory levels, reduce stockouts, and minimize excess inventory, leading to improved supply chain efficiency and cost savings.
Disruptions: Supply chains are vulnerable to various risks, including natural disasters, geopolitical tensions, transportation delays, and quality issues. A Bayesian network can be employed to assess the likelihood and impact of different risks on supply chain operations. By incorporating historical data, expert knowledge, and external factors, the network can quantify the probabilities of various risk events occurring and their potential consequences. This information can then be used to prioritize risks, develop mitigation strategies, and allocate resources effectively to minimize the impact of disruptions on the supply chain.
Supplier Selection and Contract Negotiation: When selecting suppliers and negotiating contracts, companies must consider various factors such as cost, quality, lead times, and reliability. A Bayesian network can assist in this process by modeling the probabilistic relationships between supplier attributes and performance metrics. By analyzing historical data and expert assessments, the network can calculate the probabilities of different suppliers meeting performance targets and the expected costs associated with each option. This information can help companies make informed decisions about supplier selection, contract terms, and risk allocation, ultimately leading to more robust and resilient supply chains.
These examples illustrate the versatility of Bayesian networks in addressing various challenges and opportunities in supply chain management. By leveraging probabilistic modeling and inference techniques, Bayesian networks enable companies to make data-driven decisions, enhance operational efficiency, and mitigate risks in an uncertain and dynamic environment.
2. Bayesian network for predictive maintenance
Bayesian networks can also be utilized for diagnostic and prognostic purposes in supply chain management. Here’s how they can be applied in these contexts:
Diagnostic Maintenance:
In supply chain operations, diagnostic maintenance involves identifying the root causes of failures or disruptions in machinery, equipment, or processes. Bayesian networks can aid in this process by modeling the probabilistic relationships between various factors contributing to failures and guiding diagnostic efforts.
Example: Consider a distribution center where conveyor belts are critical for material handling. A Bayesian network can be constructed to model the relationships between factors such as belt tension, motor temperature, vibration levels, conveyor speed and failures. Once a failure has happened, the maintenance crew can leverage the DAG to easily derive the potential causes that led to the failure and obtain probabilities of involvement of certain components and conditions, without even having inspected the equipment!
Prognostic Maintenance:
Prognostic maintenance involves predicting the future health and performance of machinery or equipment based on current conditions and historical data. Bayesian networks can be instrumental in prognostic maintenance by modeling degradation patterns, analyzing trends, and forecasting potential failures.
Example: In a manufacturing facility, CNC machines are critical for production. A Bayesian network can be developed to model the degradation of machine components based on factors such as operating hours, tool wear, temperature fluctuations, and vibration levels. By continuously updating the network with sensor data and historical maintenance records, operators can predict the remaining useful life of critical components, schedule proactive maintenance interventions, and avoid costly unplanned downtime.
Integrated Diagnostic and Prognostic Systems:
Bayesian networks can also be integrated into comprehensive diagnostic and prognostic systems that combine real-time monitoring, data analytics, and decision support capabilities. These systems enable proactive maintenance strategies, enhance equipment reliability, and minimize operational disruptions in the supply chain.
Example: In a logistics company managing a fleet of delivery vehicles, an integrated diagnostic and prognostic system utilizes Bayesian networks to monitor vehicle health indicators such as engine performance, fuel consumption, and tire pressure. By continuously analyzing sensor data and historical maintenance records, the system predicts potential failures, diagnoses underlying issues, and recommends maintenance actions to fleet managers. This proactive approach reduces vehicle downtime, improves fleet reliability, and ensures on-time deliveries, ultimately enhancing customer satisfaction and operational efficiency.
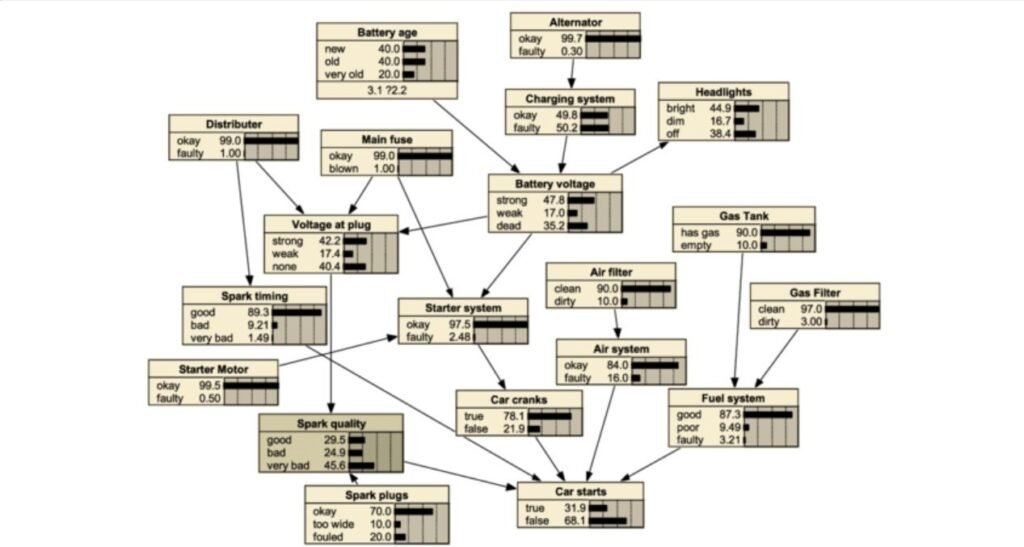
2.1 Integrating Bayesian networks with enterprise tools
Integrating a Bayesian network with a Computerized Maintenance Management System (CMMS) and Programmable Logic Controllers (PLCs) can yield significant benefits in terms of predictive maintenance, root cause analysis, and continuous improvement. Here’s how such integration can operate and the pros associated with it:
Predictive Maintenance with Bayesian Networks:
Bayesian networks can be integrated with CMMS and PLCs to predict equipment failures and maintenance needs based on real-time data and historical maintenance records. By analyzing sensor data, historical performance data, and maintenance logs, Bayesian networks can identify patterns and trends indicative of impending failures. This allows maintenance teams to proactively schedule preventive maintenance activities before equipment breakdowns occur, reducing unplanned downtime and maintenance costs.
Pros of Integration:
a. Early Detection of Failures: Bayesian networks can detect potential failures before they occur by analyzing sensor data and identifying early warning signs. This allows maintenance teams to address issues proactively, minimizing downtime and disruption to operations.
b. Root Cause Analysis: When a failure occurs, the integrated system can initiate a work order in the CMMS to conduct a deep analysis of root causes. Bayesian networks can assist in this analysis by identifying potential causes based on historical data and sensor readings. This enables maintenance teams to address underlying issues and prevent future failures.
c. Continuous Improvement through Operator Feedback: Operators play a crucial role in providing feedback to the Bayesian network about the real causes of failures. By incorporating operator input into the network, it can be continuously retrained and refined to improve its accuracy and effectiveness over time. This iterative process of learning and adaptation ensures that the network remains up-to-date and capable of accurately predicting and diagnosing equipment failures.
Example Scenario:
Let’s consider a manufacturing plant that integrates a Bayesian network with its CMMS and PLCs:
- The Bayesian network continuously monitors sensor data from PLCs installed on critical equipment, such as motors, pumps, and conveyors.
- When deviations from normal operating conditions are detected, the Bayesian network analyzes historical data and predicts potential failure modes.
- If a failure is predicted, the Bayesian network triggers a work order in the CMMS to investigate the issue further.
- Maintenance technicians use the CMMS to conduct root cause analysis, documenting their findings and actions taken.
- Operators provide feedback to the Bayesian network about the actual cause of the failure, which is used to update and refine the network.
- Over time, the Bayesian network becomes more accurate in predicting failures and identifying root causes, leading to improved equipment reliability and reduced maintenance costs.
In conclusion, integrating a Bayesian network with CMMS and PLCs offers numerous advantages in predictive maintenance, root cause analysis, and continuous improvement. By leveraging real-time data, historical records, and operator feedback, the integrated system enhances equipment reliability, minimizes downtime, and drives operational excellence in the supply chain.
01
Simulation modelling for disruption analysis and mitigation action testing
Learn how simulation modelling can support deeper understanding of disruptions and enable your business to test mitigation actions in a risk-free environment
02
Monitoring risks via probabilistic modelling and bayesian networks
Discover how to continuously monitor the risk profile thanks to an Enterprise Risk Management tool based on Bayesian Networks, Direct Acyclic Graphs and conditional probabilities
03
Monte carlo method to discover all about risks based on their severity
Estimate the potential effect of the power of chaos and randomicity on your supply chain. Empower any static risk analysis with steroids thanks to Monte carlo method