SIMULATION MODElLING AS A DECISION MAKING TOOL
WHAT IS SIMULATION MODELLING?
Simulation modelling is a sophisticated methodology for strategic planning and data-driven decision making. Picture a scenario where you’re orchestrating a complex business strategy or a complex process flow. Instead of relying solely on intuition, simulation modelling enables you to create a virtual replica of the real world in a computer. By inputting various parameters and running simulations, you can predict outcomes, identify potential bottlenecks, and optimize processes. It’s akin to a virtual rehearsal, providing a nuanced understanding of how different variables interact in complex systems.
To learn how advanced such methodologies are and to understand the extent of support that they can offer to decision-making processes, we can map them on the analytics pyramid.
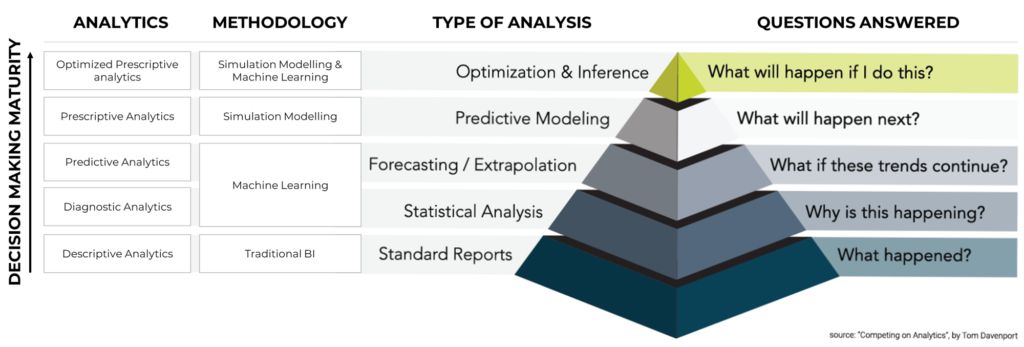
The journey from descriptive to prescriptive analytics is closely intertwined with the adoption and integration of advanced technologies such as simulation modelling, machine learning, business intelligence (BI) tools, and digital twins. These technologies play pivotal roles in enhancing the capabilities of each analytical stage, contributing to a more comprehensive and insightful decision-making process.
1. Descriptive Analytics and BI Tools:
Descriptive analytics, with its focus on summarizing historical data, is greatly facilitated by BI tools. These tools enable organizations to visually explore and interpret data trends through intuitive dashboards and reports.
Business intelligence platforms provide an interactive and user-friendly interface for stakeholders to analyze and understand the past, facilitating data-driven insights that are crucial for strategic planning and performance evaluation.
2. Diagnostic Analytics and Machine Learning:
Diagnostic analytics involves the examination of historical data to understand the reasons behind past events or outcomes. It aims to identify patterns, correlations, and causation within the data. For instance, in a business context, diagnostic analytics might explore why sales dropped during a specific period or why certain marketing strategies were more effective than others.
3. Predictive Analytics and Machine Learning:
Predictive analytics, essential for anticipating future trends, heavily relies on machine learning algorithms. Machine learning enables organizations to build predictive models that learn from historical data patterns and make accurate forecasts. The dynamic nature of machine learning algorithms allows businesses to adapt to changing environments and make data-driven predictions, enhancing decision-making in areas such as demand forecasting, resource optimization, and risk management.
4. Prescriptive Analytics, Simulation Modelling and Digital Twins:
At the apex of the analytical spectrum, prescriptive analytics is further strengthened by the concept of digital twins.
A digital twin is a virtual representation of a physical system or process. They enable organizations to simulate different scenarios in a virtual environment to make impact-driven decisions. By closely mirroring real-world conditions, digital twins provide a platform for testing and refining prescriptive recommendations, ensuring that actions are tested in a risk-free environment and are based on accurate and up-to-date information.
5. Optimized Prescriptive Analytics, Simulation Modelling and Machine Learning:
To evaluate various decision options and recommend the most advantageous course of action, considering constraints and objectives, simulation modelling and machine learning can be applied.
Simulation Modeling enables the representation of complex systems through virtual scenarios, providing a dynamic environment to test and analyze different strategies.
Machine Learning, on the other hand, empowers systems to learn from data and make predictions, enhancing the decision-making process with predictive insights. Together, these technologies synergize to create a comprehensive framework that not only diagnoses current situations but also prescribes optimal solutions, while constantly adapting and improving based on real-time feedback. This integration facilitates proactive decision-making, fosters efficiency, and propels organizations toward more agile and intelligent operations.
1. Descriptive Analytics and BI Tools
Descriptive analytics, with its focus on summarizing historical data, is greatly facilitated by BI tools. These tools enable organizations to visually explore and interpret data trends through intuitive dashboards and reports.
Business intelligence platforms provide an interactive and user-friendly interface for stakeholders to analyze and understand the past, facilitating data-driven insights that are crucial for strategic planning and performance evaluation.
2. Diagnostic Analytics and Machine Learning
Diagnostic analytics involves the examination of historical data to understand the reasons behind past events or outcomes. It aims to identify patterns, correlations, and causation within the data. For instance, in a business context, diagnostic analytics might explore why sales dropped during a specific period or why certain marketing strategies were more effective than others.
3. Predictive Analytics and Machine Learning
Predictive analytics, essential for anticipating future trends, heavily relies on machine learning algorithms. Machine learning enables organizations to build predictive models that learn from historical data patterns and make accurate forecasts. The dynamic nature of machine learning algorithms allows businesses to adapt to changing environments and make data-driven predictions, enhancing decision-making in areas such as demand forecasting, resource optimization, and risk management.
4. Prescriptive Analytics, Simulation Modelling and Digital Twins
At the apex of the analytical spectrum, prescriptive analytics is further strengthened by the concept of digital twins.
A digital twin is a virtual representation of a physical system or process. They enable organizations to simulate different scenarios in a virtual environment to make impact-driven decisions. By closely mirroring real-world conditions, digital twins provide a platform for testing and refining prescriptive recommendations, ensuring that actions are tested in a risk-free environment and are based on accurate and up-to-date information.
5. Optimized Prescriptive Analytics, Simulation Modelling and Machine Learning
To evaluate various decision options and recommend the most advantageous course of action, considering constraints and objectives, simulation modelling and machine learning can be applied.
Simulation Modeling enables the representation of complex systems through virtual scenarios, providing a dynamic environment to test and analyze different strategies.
Machine Learning, on the other hand, empowers systems to learn from data and make predictions, enhancing the decision-making process with predictive insights. Together, these technologies synergize to create a comprehensive framework that not only diagnoses current situations but also prescribes optimal solutions, while constantly adapting and improving based on real-time feedback. This integration facilitates proactive decision-making, fosters efficiency, and propels organizations toward more agile and intelligent operations.
In essence, the synergy between all the 5 approaches to analytics creates a powerful analytical ecosystem.
BI tools empower descriptive analytics, machine learning drives predictive analytics, simulation modeling and digital twins enhances prescriptive analytics, while by combining the most advanced methodologies, it is possible to achieve the very edge of supply chain operational excellence. As organizations embrace these technologies in tandem with their analytical journey, they gain a holistic and data-driven approach to decision-making, enabling them to navigate complexities and uncertainties with agility and precision.
To learn more about the advanced approaches, we suggest 6 papers to deep dive on the cutting-edge technologies and methodologies of simulation modelling and digital twins
01
Problem solving in supply chain processes
Problem by problem: Analytical methods and Dynamic Simulation compared
02
Dynamic simulation as decision support
How to choose the right simulation methodology for a tailor made approach to reliable data-driven decision
04
Skills and technologies
Learn about the skills and technologies involved in this cutting-edge technology
06
Applying Simulation to the S&OP process
MRP, Scheduling, and in between Simulation Modelling: discover how